As a data/product analyst, your team often relies upon you as an unbiased source of truth and you rely on your credibility to influence through your recommendations. However, as everyone working with data knows, biases are everywhere. There are unknowable answers, the same data can be interpreted in various ways, and biases are everywhere!
Sometimes, while I lie awake at night, I can’t stop thinking how many analytics professionals might be misleading teams with average levels of data literacy to put too much faith in numbers or interpretations that have various degrees of certainty. It’s a terrifying thought because one of the first things every data analyst, product manager, should do is to gain the trust of their team.
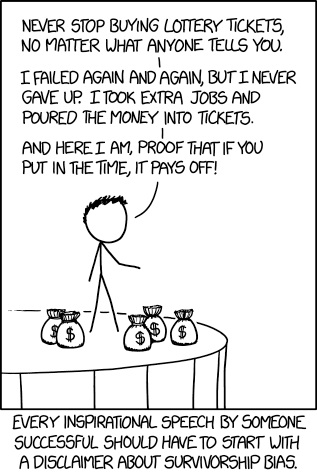
We need to create this trusting relationship so that we can communicate insights, and influence teams to take action. It’s important that you are trustworthy, and your recommendations are made with the intent to improve your product. Otherwise, you wouldn’t be behaving ethically! However, all analyses and interpretations have varying degrees of uncertainty attached to them, and misunderstandings and miscommunications might affect your credibility.
Your recommendations are more often than not based on more than just the pure facts in front of you. The core of the analyst work is in the interpretation; the way you weave together observations and storytelling.
How can analysts improve their trustworthiness and credibility?
I can see a few points that can strengthen any team that works with analytics and their relationship with analysts or other data professionals:
- Investing in improving the data literacy of a team:
- Teach statistics 101 and keep refreshing that knowledge periodically.
- Walk them through your thought process/reasoning.
- Discuss uncertainty, and get the team comfortable with probability, and risk.
- Be aware when you’re using the “analyst hat”, and make sure your audience knows whether they’re listening to a fact, an interpretation based on strong evidence, a plain “gut-feeling hypothesis”, or anything in between.
- Discuss and peer-review your work with other analysts.
- Be curious about interpretations coming from less experienced analysts, because these might make you aware of some of your own biases.
Final Reflections on Data-Driven Teams
Heading towards 2022, it might be time to switch from “data-driven” development to “hypothesis-driven” development. It’s time to leave the old over-reliant faith in numbers and explore a more human-centric/scientific way of making sense of the world around us. Acknowledging uncertainty is not a weakness but a strength that improves the decision-making process.
I find that the more experience I get helping teams make sense of their data, the more I look at systems holistically and the more baggage of product knowledge I accrue, and the more I trust my gut feeling. I don’t think I’m alone in exploring these interesting sides of decision-making, a former colleague wrote a wonderful post on the importance of intuition in the decision-making process (Escaping a killer shark with a rushed decision — Intuition: The logical paradox). This means that communication with stakeholders has to be as clear as possible, and it’s important to be introspective and reflect on personal/systematic biases.
These are some reflections on professional credibility when working with data in a support function. I would love to hear more feedback and comments about it.
- Do you agree with these points?
- Have you ever felt that your biases were affecting the quality of your recommendations negatively?
- Do you have any stories of how your “product baggage” has helped you steer a team into a successful outcome?
- Have you been with a team long enough to reap the benefits of investing in their data literacy?
If you’re interested in becoming a Data Analyst, check out Breaking into the Games Industry as a Game Analyst.
Pingback: Improve usage of acronyms, a New Year's wish - Daantje on Data